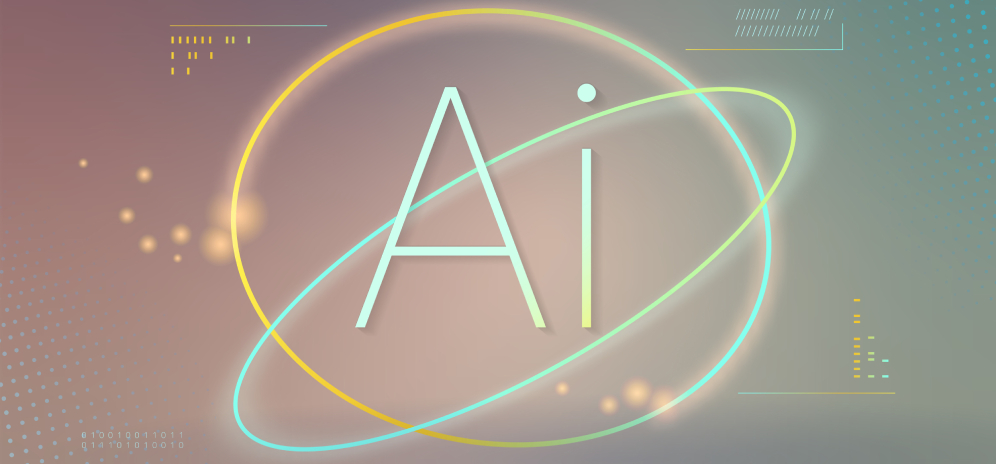
In recent years, artificial intelligence (AI) has made remarkable strides, particularly in the field of generative models. These sophisticated algorithms are designed to generate new and original content, ranging from images and music to text and even videos. Harnessing the power of deep learning, these generative AI models have revolutionized various industries, offering unprecedented possibilities for creativity and innovation. In this blog post, we will delve into the captivating world of generative AI models, exploring their capabilities, applications, and potential impact on our society.
Understanding Generative AI Models
Generative AI models are a subset of machine learning algorithms that learn to create new data that resembles a given dataset. They leverage complex neural networks and vast amounts of training data to generate output that exhibits patterns and characteristics similar to the input data. These models can be broadly categorized into two types: Variational Autoencoders (VAEs) and Generative Adversarial Networks (GANs).
- Variational Autoencoders (VAEs): VAEs are generative models that learn the underlying distribution of a dataset and generate new samples based on that distribution. They consist of two main components: an encoder and a decoder. The encoder compresses the input data into a lower-dimensional representation, known as a latent space, while the decoder reconstructs the original data from the latent space. By sampling from the latent space, VAEs can generate novel outputs that resemble the training data.
- Generative Adversarial Networks (GANs): GANs are another class of generative models that involve a dynamic interplay between two neural networks: a generator and a discriminator. The generator creates synthetic data samples, while the discriminator tries to distinguish between real and generated samples. Through an adversarial training process, the generator learns to improve its output, while the discriminator becomes more adept at distinguishing real from fake data. GANs have been remarkably successful in generating realistic images, videos, and audio.
Applications of Generative AI Models
The applications of generative AI models span across multiple domains, offering exciting possibilities for creative expression, problem-solving, and entertainment. Here are some notable areas where these models are making a significant impact:
- Creative Design: Generative AI models can aid artists, designers, and architects in generating novel and inspiring creations. They can produce unique artwork, generate virtual environments, and even design novel product prototypes.
- Content Generation: Text generation models can produce coherent and contextually relevant written content, assisting writers, journalists, and content creators. They can be used for automated content creation, summarization, and translation.
- Visual Media: Generative models can generate realistic images, modify existing images, and even create novel visual concepts. They have potential applications in gaming, animation, film production, and virtual reality experiences.
- Music and Audio: AI models can compose original music, generate realistic instrument sounds, and even mimic the style of famous musicians. They have been used in music production, sound design, and creating personalized soundtracks.
- Healthcare and Research: Generative models have demonstrated utility in medical imaging, drug discovery, and disease diagnosis. They can generate synthetic medical images, simulate biological systems, and aid in analyzing complex data sets.
Ethical Considerations and Challenges
While generative AI models offer tremendous possibilities, they also raise important ethical considerations and challenges. Issues related to intellectual property, data privacy, and bias in generated content need careful attention. Ensuring responsible use of these models and understanding their limitations is crucial to prevent misuse and potential harm.
Conclusion
Generative AI models have emerged as powerful tools for creativity, innovation, and problem-solving across various industries. Their ability to generate original and realistic content has transformed the way we approach creative endeavors, offering new avenues for artistic expression and pushing the boundaries of what is possible. From generating stunning visuals to composing music that stirs the soul, these models have demonstrated their potential to augment human creativity.
However, as we continue to explore the capabilities of generative AI models, it is essential to remain mindful of the ethical considerations they raise. One of the primary concerns is the issue of intellectual property. When AI generates content, who owns the rights to that content? Determining the boundaries and legal frameworks for ownership and attribution can be complex and requires careful consideration.
Another important consideration is data privacy. Generative AI models often require extensive datasets for training, and ensuring that these datasets are collected and used ethically is crucial. Privacy concerns must be addressed to protect individuals’ personal information and prevent the misuse of data.
Bias in generated content is another significant challenge. AI models learn from the data they are trained on, and if the training data contains biases or prejudices, those biases may be reflected in the generated output. Recognizing and mitigating bias in generative AI models is essential to ensure fair and inclusive content generation.
Moreover, the potential misuse of generative AI models raises concerns about misinformation, forgery, and malicious intent. As these models become more sophisticated, the risk of generating convincing fake content increases. This calls for the development of robust detection mechanisms and responsible use guidelines to safeguard against harmful applications.
Despite these challenges, the future of generative AI models is undeniably exciting. Continued research and innovation in this field hold immense promise for creative industries, scientific advancements, and societal progress. However, it is crucial to navigate this landscape with caution and an ethical framework that ensures the responsible use and development of these models.
In conclusion, generative AI models have revolutionized the way we think about creativity, pushing the boundaries of what can be achieved. From generating stunning visuals and composing music to aiding in healthcare and research, these models offer a world of possibilities. However, ethical considerations must remain at the forefront of their development and use. By addressing issues related to intellectual property, data privacy, bias, and misuse, we can harness the potential of generative AI models to create a more inclusive and responsible future. Let us embrace this technological marvel while being mindful of the ethical responsibilities it brings. The world of generative AI is full of wonder and endless possibilities, and by navigating it responsibly, we can unlock new frontiers of creativity and innovation.
Recent Comments